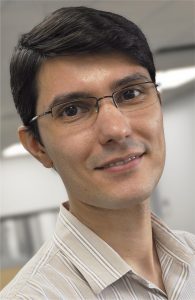
Rogers Silva
Research Scientist
TReNDS Center & Georgia State University
Dr. Rogers F. Silva is a multidisciplinary scientist with extensive experience developing algorithms for statistical and machine learning, image analysis, numerical optimization, memory-efficient large-scale data reduction, and distributed analyses of big multimodal, multi-subject neuroimaging data.
He received the B.Sc. degree in Electrical Engineering in 2003 from the Catholic University (PUCRS), Porto Alegre, Brazil, the M.S. degree in Computer Engineering (with minors in Statistics and in Mathematics) in 2011, and the Ph.D. degree (with distinction) in Computer Engineering in 2017, both from The University of New Mexico, Albuquerque, NM, USA. He also received training in diffusion spectrum imaging at CMU and University of Pittsburgh in 2016, multimodal brain imaging at the MGH Martinos Center and Harvard/MIT in 2016, and deep learning at MILA, University of Montreal in 2017.
Before joining the TReNDS Center, Dr. Silva developed novel approaches for multimodal medical image analysis as a Postdoctoral Fellow at the Mind Research Network, in addition to client-centric tools and processes using machine learning and statistical analysis as a Data Scientist with Datalytic Solutions. Previously, he also had experience as an engineer, lecturer, and consultant.
As a modeling-oriented scientist actively conducting research on novel multimodal multidimensional learning (MML) methods for brain research, Dr. Silva leverages his multidisciplinary background to create new algorithms for deep unsupervised learning that can fully leverage the joint information and shared variability contained in heterogeneous multimodal (or multiview) datasets. He also develops new algorithms for federated learning of private multi-site data, seeking to enable collaborative research that can take advantage of decentralized datasets without requiring direct access to data stored in remote data centers.
His research interests include
· heterogeneous multimodal data fusion
· deep statistical and machine learning
· image, video and data analysis
· multiobjective, combinatorial and constrained optimization
· image and signal processing
· multimodal neuroimaging